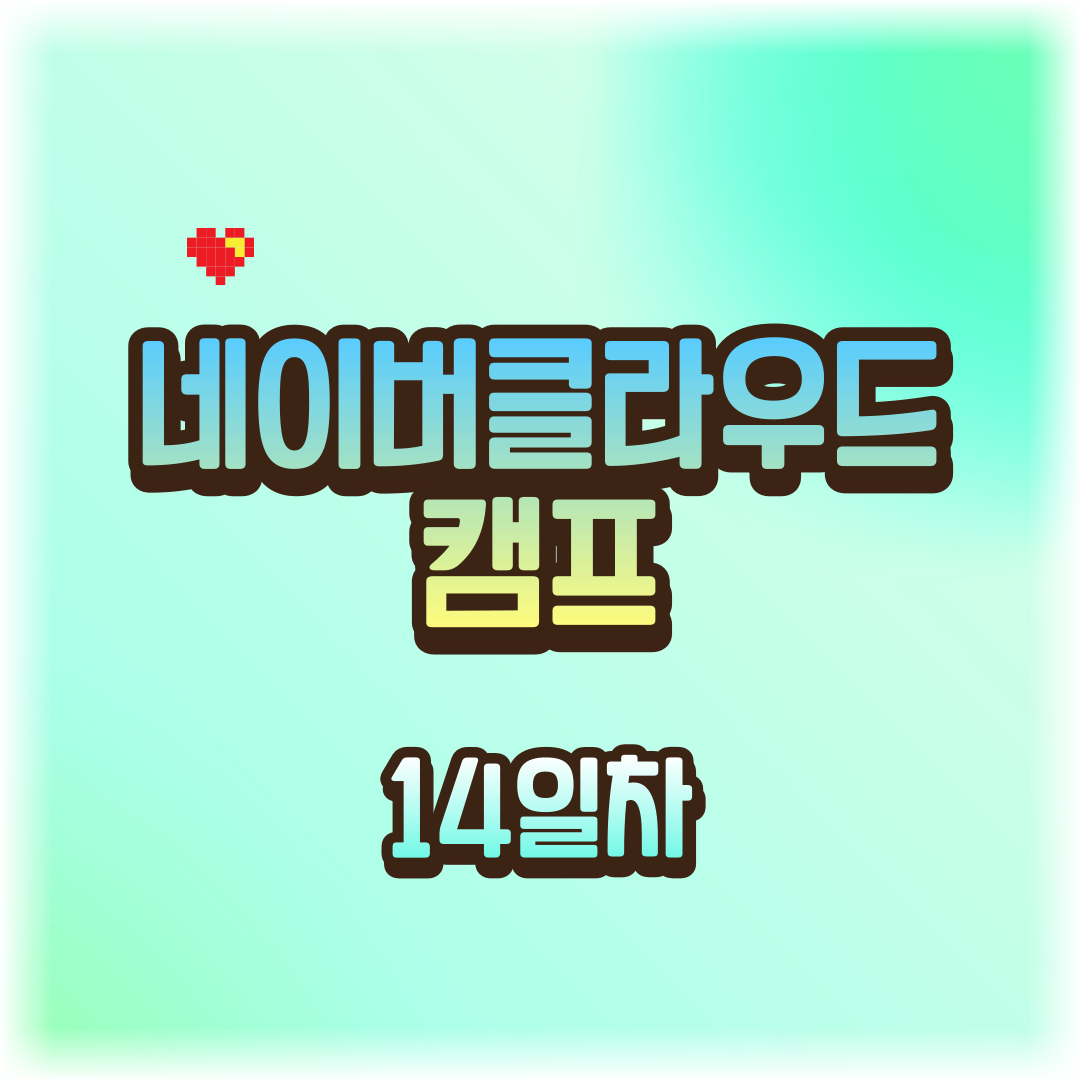
자연어처리(NLP) 기초
1. 워드 임베딩 x (Word Embedding)
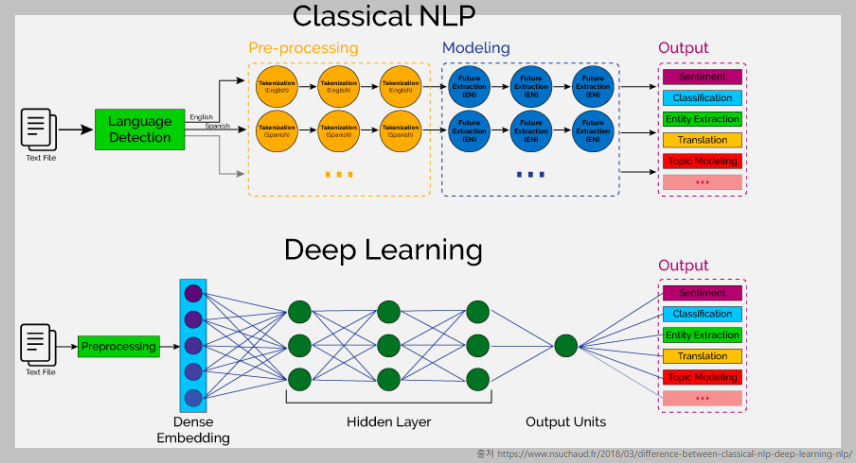
- 텍스트를 컴퓨터가 이해할 수 있도록 숫자로 변환
- 단어를 표현하는 방법에 따라서 자연어 처리의 성능이 크게 달라짐
- 워드 임베딩은 각 단어를 인공 신경망 학습을 통해 벡터(Vector)화하는 방법
- 케라스에서 제공하는 Embedding() => 단어를 랜덤한 값을 가지는 벡터로 변환한 뒤에,
인공 신경망의 가중치를 학습
2. 인공지능에서 벡터 x (vector)란

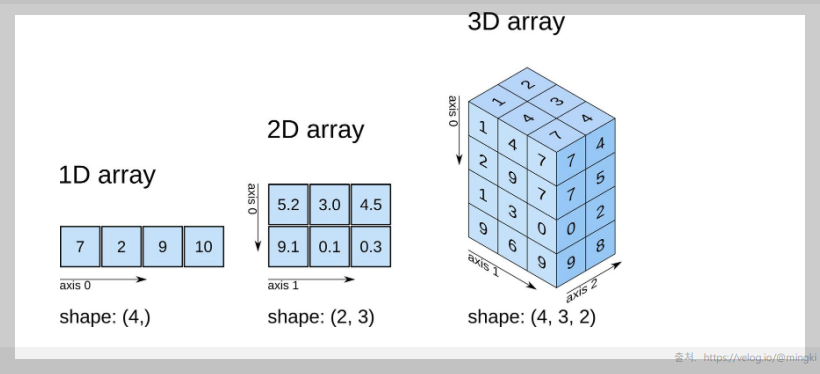
vector
(50000,32,32,3)
60000,28,28) # reshape가 필요한 형태
이러한 형태를 '벡터'라고함.
- 인공지능 분야에서 벡터는 대개 고차원의 숫자 배열(array)을 의미함
- 이미지 데이터의 벡터는 각 픽셀(pixel)의 색상 값을 숫자로 표현하고,
이러한 숫자들을 배열 형태로 나열한 것 (예를 들어, 28 x 28 픽셀의 흑백 이미지는 784차원의 벡터)
- 텍스트 데이터의 벡터는 각 단어(word)를 고유한 정수 인덱스(index)로 매핑하여,
이를 순서대로 배열한 것 단어 간의 유사도 계산, 문서 간의 유사도 비교 등 다양하게 활용
3. 케라스 Embedding()

케라스의 Embedding() - 훈련 데이터의 단어들에 대해 워드 임베딩을 수행
tensorflow.keras tokenizer - 문장의 감성 분류 모델
케라스의 Embedding() - 훈련 데이터의 단어들에 대해 워드 임베딩을 수행
import numpy as np
from tensorflow.keras.preprocessing.text import Tokenizer
from tensorflow.keras.preprocessing.sequence import pad_sequences
sentences = ['nice great best amazing', 'stop lies', 'pitiful nerd’,
'excellent work', 'supreme quality', 'bad', 'highly respectable’]
y_train = [1, 0, 0, 1, 1, 0, 1] 긍정인 문장은 1, 부정인 문장은 0
문장의 긍, 부정을 판단하는 감성 분류 모델
1. keras의 tokenizer를 사용하여 단어 집합을 만들고 크기 확인
tokenizer = Tokenizer()
tokenizer.fit_on_texts(sentences)
vocab_size = len(tokenizer.word_index)
2. 각 문장에 대해서 정수 인코딩을 수행
X_encoded = tokenizer.texts_to_sequences(sentences)
3. 가장 길이가 긴 문장의 길이 계산
max_len = max(len(l) for l in X_encoded)
4. 최대 길이로 모든 샘플에 대해서 패딩 진행
X_train = pad_sequences(X_encoded, maxlen=max_len, padding='post’)
y_train = np.array(y_train)
print('패딩 결과 :’)
print(X_train)
5. 전형적인 이진 분류 모델을 설계
from tensorflow.keras.models import Sequential
from tensorflow.keras.layers import Dense, Embedding, Flatten
embedding_dim = 4
model = Sequential()
model.add(Embedding(vocab_size, embedding_dim, input_length=max_len))
model.add(Flatten())
model.add(Dense(1, activation='sigmoid')) => output에 1개의 뉴런을 배치 활성화 함수는 시그모이드 함수
model.compile(optimizer='adam', loss='binary_crossentropy', metrics=['acc'])
model.fit(X_train, y_train, epochs=100, verbose=2) => 손실 함수로 binary_crossentropy 사용
[실습코드]
import numpy as np
from keras.preprocessing.text import Tokenizer
# 1. 데이터
docs = ['재밌어요', '재미없다', '돈 아깝다', '숙면했어요',
'최고에요', '꼭봐라', '세번 봐라','또보고싶다','n회차 관람',
'배우가 잘생기긴했다','발연기', '추천해요', '최악','후회된다',
'돈버렸다', '글쎄요','보다 나왔다','망작이다','연기가 어색',
'차라리 기부가 낫다', '후속작 만들어라', '역시 전작이 낫다',
'왜그랬대', '다른거 볼걸', '감동이다']
# 긍정 1, 부정 0
labels = np.array([1, 0, 0, 0, 1,1,1,1,1,1,0,1,0,0,0,0,0,0,0,0,0,0,0,1,1])
token = Tokenizer()
token.fit_on_texts(docs)
# print(token.word_index)
'''
#{'낫다': 1, '재밌어요': 2, '재미없다': 3, '돈': 4, '아깝다': 5, '숙면했어요': 6,
# '최고에요': 7, '꼭봐라': 8,
# '세번': 9, '봐라': 10, '또보고싶다': 11, 'n회차': 12, '관람': 13,
# '배우가': 14, '잘생기긴했다': 15, '발연기': 16, ' 추천해요': 17,
# '최악': 18, '후회된다': 19, '돈버렸다': 20, '글쎄요': 21,
# '보다': 22, '나왔다': 23, '망작이다': 24, '연기가': 25,
# '어색': 26, '차라리': 27, '기부가': 28, '후속작': 29,
# '만들어라': 30, '역시': 31, '전작이': 32, '왜그랬대': 33,
# '다른거': 34, '볼걸': 35, '감동이다': 36}
'''
x = token.texts_to_sequences(docs)
#print(x)
'''
#[[2], [3], [4, 5], [6], [7], [8], [9, 10], [11], [12, 13], [14, 15],
# [16], [17], [18], [19], [20], [21], [22, 23], [24], [25, 26],
# [27, 28, 1], [29, 30], [31, 32, 1], [33], [34, 35], [36]]
'''
# pad_sequences
from keras_preprocessing.sequence import pad_sequences
pad_x = pad_sequences(x, padding='pre', maxlen=3)
print(pad_x.shape) # (25, 3)
word_size = len(token.word_index)
print('word_size : ', word_size) # word_size : 36
# 모델
from keras.models import Sequential
from keras.layers import Dense, Embedding, LSTM
model = Sequential()
model.add(Embedding(input_dim = 37, # word_size : 36 + 1
output_dim = 10, # node 수
input_length=3)) # Max_문장의 길이
model.add(LSTM(32))
model.add(Dense(1, activation='sigmoid')) # sigmoid 이진분류이기 때문.
# model.summary()
# 컴파일, 훈련
model.compile(loss='binary_crossentropy',
optimizer='adam',
metrics='acc')
model.fit(pad_x, labels, epochs=100, batch_size=16)
#4. 평가, 예측
loss, acc = model.evaluate(pad_x, labels)
print('loss : ', loss)
print('acc : ', acc)
#loss : 0.10301996022462845
#acc : 1.0
# predict
#x_predict = '정말 정말 재미있고 최고에요'
x_predict = '영화가 정말 재미없네'
# 1. Tokenization
token_pre = Tokenizer()
x_predict = np.array([x_predict])
print(x_predict)
token_pre.fit_on_texts(x_predict)
x_pred = token_pre.texts_to_sequences(x_predict)
print(token_pre.word_index)
print(x_pred)
# 2) Padding
x_pred = pad_sequences(x_pred, padding='pre')
print(x_pred)
# 3) Model Prediction
y_pred = model.predict(x_pred)
print(y_pred)
https://dev-with-gpt.tistory.com/55
파이썬 딥러닝 자연어처리 실습 (Tokenizer, Embedding, Rueters,Imdb)
1. Tokenizer [설명] Tokenizer는 자연어 처리에서 텍스트를 분석할 수 있는 형태, 주로 '토큰(token)'이라는 단위로 분리하는 작업을 수행하는 도구입니다. '토큰'은 문맥에 따라 다르지만, 대부분의 경
dev-with-gpt.tistory.com
1. Tokenizer

[설명]
Tokenizer는 자연어 처리에서 텍스트를 분석할 수 있는 형태, 주로 '토큰(token)'이라는 단위로 분리하는 작업을 수행하는 도구입니다. '토큰'은 문맥에 따라 다르지만, 대부분의 경우 단어, 문장, 문단 등을 의미합니다.
토큰화의 주 목적은 원시 텍스트 데이터를 머신러닝 알고리즘이 처리할 수 있는 구조로 변환하는 것입니다.
[코드]
from keras.preprocessing.text import Tokenizer
text = '나는 진짜 매우 매우 매우 매우 맛있는 밥을 엄청 많이 많이 많이 먹어서 매우 배가 부르다.'
token = Tokenizer()
token.fit_on_texts([text]) # fit on 하면서 index 생성됨
index = token.word_index
x = token.texts_to_sequences([text])
print(x)
print(token.index_word)
[출력]
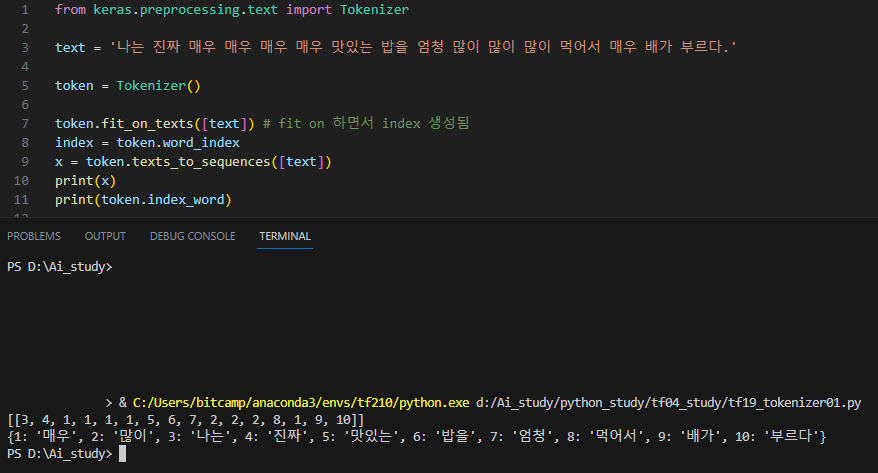
2. Embedding

[설명]
입력 레이어(임베딩)
input_dim=37: 입력 단어 인덱스 범위는 0에서 36까지입니다(36이 최대 단어 인덱스임).
output_dim=10: 각 단어 색인은 차원 10의 조밀한 벡터로 표현됩니다.
input_length=3: 입력 시퀀스는 3개의 워드 인덱스로 구성됩니다.
숨겨진 계층은 LSTM 계층으로, 순차적 정보를 캡처하도록 설계되었습니다.
이 레이어에 지정된 매개변수는 다음과 같습니다.
히든 레이어(LSTM)
LSTM(32): 레이어에는 각 시간 단계에서 32개의 LSTM 단위(또는 메모리 셀)가 있습니다.
출력 레이어는 단일 뉴런과 이진 분류를 가능하게 하는 시그모이드 활성화 함수가 포함된 Dense 레이어입니다. 이 레이어에 지정된 매개변수는 다음과 같습니다.
출력 레이어(시그모이드 활성화로 밀도가 높음)
Dense(1, activation='sigmoid'): 출력 레이어는 입력을 기반으로 정서(긍정적 또는 부정적)를 예측하고 Sigmoid 활성화 함수는 출력이 0과 1 사이가 되도록 합니다.
전체 모델 아키텍처는 다음과 같이 요약할 수 있습니다.
임베딩 레이어는 (batch_size, input_length, output_dim)입력 길이와 출력 치수에 따라 모양이 있는 3D 텐서를 출력합니다.
LSTM 레이어는 지정된 LSTM 단위 수에 따라 모양이 있는 2D 텐서를 출력합니다 .
출력의 Dense 레이어는 (batch_size, 1)이진 분류 예측을 나타내는 모양을 가진 2D 텐서를 생성합니다.
[코드]
import numpy as np
from keras.preprocessing.text import Tokenizer
# 1. 데이터
docs = ['재밌어요', '재미없다', '돈 아깝다', '숙면했어요',
'최고에요', '꼭봐라', '세번 봐라','또보고싶다','n회차 관람',
'배우가 잘생기긴했다','발연기', '추천해요', '최악','후회된다',
'돈버렸다', '글쎄요','보다 나왔다','망작이다','연기가 어색',
'차라리 기부가 낫다', '후속작 만들어라', '역시 전작이 낫다',
'왜그랬대', '다른거 볼걸', '감동이다']
# 긍정 1, 부정 0
labels = np.array([1, 0, 0, 0, 1,1,1,1,1,1,0,1,0,0,0,0,0,0,0,0,0,0,0,1,1])
token = Tokenizer()
token.fit_on_texts(docs)
# print(token.word_index)
'''
#{'낫다': 1, '재밌어요': 2, '재미없다': 3, '돈': 4, '아깝다': 5, '숙면했어요': 6,
# '최고에요': 7, '꼭봐라': 8,
# '세번': 9, '봐라': 10, '또보고싶다': 11, 'n회차': 12, '관람': 13,
# '배우가': 14, '잘생기긴했다': 15, '발연기': 16, ' 추천해요': 17,
# '최악': 18, '후회된다': 19, '돈버렸다': 20, '글쎄요': 21,
# '보다': 22, '나왔다': 23, '망작이다': 24, '연기가': 25,
# '어색': 26, '차라리': 27, '기부가': 28, '후속작': 29,
# '만들어라': 30, '역시': 31, '전작이': 32, '왜그랬대': 33,
# '다른거': 34, '볼걸': 35, '감동이다': 36}
'''
x = token.texts_to_sequences(docs)
#print(x)
'''
#[[2], [3], [4, 5], [6], [7], [8], [9, 10], [11], [12, 13], [14, 15],
# [16], [17], [18], [19], [20], [21], [22, 23], [24], [25, 26],
# [27, 28, 1], [29, 30], [31, 32, 1], [33], [34, 35], [36]]
'''
# pad_sequences
from keras_preprocessing.sequence import pad_sequences
pad_x = pad_sequences(x, padding='pre', maxlen=3)
print(pad_x.shape) # (25, 3)
word_size = len(token.word_index)
print('word_size : ', word_size) # word_size : 36
# 모델
from keras.models import Sequential
from keras.layers import Dense, Embedding, LSTM
model = Sequential()
model.add(Embedding(input_dim = 37, # word_size : 36 + 1
output_dim = 10, # node 수
input_length=3)) # Max_문장의 길이
model.add(LSTM(32))
model.add(Dense(1, activation='sigmoid')) # sigmoid 이진분류이기 때문.
# model.summary()
# 컴파일, 훈련
model.compile(loss='binary_crossentropy',
optimizer='adam',
metrics='acc')
model.fit(pad_x, labels, epochs=100, batch_size=16)
#4. 평가, 예측
loss, acc = model.evaluate(pad_x, labels)
print('loss : ', loss)
print('acc : ', acc)
#loss : 0.10301996022462845
#acc : 1.0
# predict
#x_predict = '정말 정말 재미있고 최고에요'
x_predict = '영화가 정말 재미없네'
# 1. Tokenization
token_pre = Tokenizer()
x_predict = np.array([x_predict])
print(x_predict)
token_pre.fit_on_texts(x_predict)
x_pred = token_pre.texts_to_sequences(x_predict)
print(token_pre.word_index)
print(x_pred)
# 2) Padding
x_pred = pad_sequences(x_pred, padding='pre')
print(x_pred)
# 3) Model Prediction
y_pred = model.predict(x_pred)
print(y_pred)
[출력]
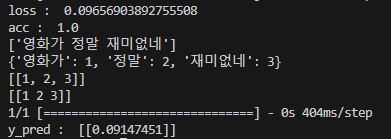
3. Embedding - Rueters
[코드]
import numpy as np
from keras.models import Sequential
from keras.layers import Dense, Embedding, LSTM, Dropout
from keras.datasets import imdb
from keras.preprocessing.text import Tokenizer
from keras_preprocessing.sequence import pad_sequences
from keras.datasets import reuters
from sklearn.metrics import r2_score, accuracy_score
import time
#pip install numpy==1.19.5
#import numpy as np
# 1. 데이터
(x_train, y_train), (x_test, y_test) = reuters.load_data(
num_words=10000) # 가져오려는 데이터의 숫자를 지정함.
print(x_train.shape, y_train.shape)
print(x_test.shape, y_test.shape)
# 최대길이와 평균길이
print('리뷰의 최대 길이 : ', max(len(i) for i in x_train))
print('리뷰의 평균 길이 : ', sum(map(len, x_train )) / len(x_train))
num_classes = len(np.unique(y_train))
print("클래스의 수:", num_classes)
max_length = 2376
#x_train = pad_sequences(x_train, maxlen=max_length)
#x_test = pad_sequences(x_test, maxlen=max_length)
x_train = pad_sequences(x_train, padding='pre', maxlen=100)
x_test = pad_sequences(x_test, padding='pre', maxlen=100)
print(x_train.shape, y_train.shape)
print(x_test.shape, y_test.shape)
model = Sequential()
model.add(Embedding(input_dim = 10000, output_dim=100)) # 데이터를 '10000'으로 설정했음
model.add(LSTM(128, activation = 'relu'))
model.add(Dropout(0.25))
model.add(Dense(256, activation = 'relu'))
model.add(Dropout(0.25))
model.add(Dense(256, activation = 'relu'))
model.add(Dropout(0.25))
model.add(Dense(256, activation = 'relu'))
model.add(Dropout(0.25))
model.add(Dense(256, activation = 'relu'))
model.add(Dropout(0.25))
model.add(Dense(46, activation = 'softmax'))
model.compile(loss='sparse_categorical_crossentropy',
optimizer='adam',
metrics='acc')
start_time = time.time()
model.fit(x_train, y_train, epochs=26, batch_size=512, validation_split=0.4)
end_time = time.time() - start_time
loss, acc = model.evaluate(x_train, y_train)
print('loss : ', loss)
print('acc : ', acc)
[출력]

Rueters. 데이터를 로드하여 출력하여 acc 를 예측하였다. 강사님께서 0.5보다 높으면 매우 좋은 정확도가 나온 것이라고 해주셨다. 사실 이 acc는 내가 인위적으로 acc를 높게 설정하려고 해서 loss 값과 acc 값이 높게 나온 것이다. 노드 수를 늘리고 Dropout을 많이 주어서 시간을 빠르게 하고 acc 값만을 높이려 하였다.
4. Embedding - Imdb
[코드]
import numpy as np
from keras.models import Sequential
from keras.layers import Dense, Embedding, LSTM, Dropout
from keras.datasets import imdb
from keras.preprocessing.text import Tokenizer
from keras_preprocessing.sequence import pad_sequences
import time
# 1. 데이터
(x_train, y_train), (x_test, y_test) = imdb.load_data(
num_words=10000) # 가져오려는 데이터의 숫자를 지정함.
print(x_train.shape, y_train.shape)
print(x_test.shape, y_test.shape)
# 최대길이와 평균길이
print('리뷰의 최대 길이 : ', max(len(i) for i in x_train))
print('리뷰의 평균 길이 : ', sum(map(len, x_train )) / len(x_train))
# 2. 모델구성
model = Sequential()
model.add(Embedding(input_dim = 10000, output_dim=100)) # 데이터를 '10000'으로 설정했음
model.add(LSTM(128, activation = 'relu'))
model.add(Dropout(0.25))
model.add(Dense(64, activation = 'relu'))
model.add(Dropout(0.25))
model.add(Dense(64, activation = 'relu'))
model.add(Dropout(0.25))
model.add(Dense(1, activation = 'sigmoid'))
model.compile(loss='binary_crossentropy',
optimizer='adam',
metrics='acc')
# 3. 데이터 전처리
# 텍스트 데이터를 숫자로 변환하기 위해 Tokenizer를 사용합니다.
tokenizer = Tokenizer(num_words=10000)
x_train = tokenizer.sequences_to_matrix(x_train, mode='binary')
x_test = tokenizer.sequences_to_matrix(x_test, mode='binary')
# 입력 데이터의 길이를 맞추기 위해 패딩을 적용합니다.
max_length = 500
x_train = pad_sequences(x_train, maxlen=max_length)
x_test = pad_sequences(x_test, maxlen=max_length)
# 4. 모델 훈련
from keras.callbacks import EarlyStopping, ModelCheckpoint
earlyStopping = EarlyStopping(monitor='val_loss', patience=50, mode='min',
verbose=1, restore_best_weights=True)
from keras.callbacks import ModelCheckpoint
# Model Check Point
mcp = ModelCheckpoint(
filepath='./_mcp/t_california.hdf5',
monitor='val_loss',
mode='auto',
verbose=1,
save_best_only=True,
save_weights_only=False
)
start_time = time.time()
model.fit(x_train, y_train, epochs=10, batch_size=32, validation_split=0.2)
end_time = time.time() - start_time
# 5. 모델 평가
loss, accuracy = model.evaluate(x_test, y_test)
print("Test Loss:", loss)
print("Test Accuracy:", accuracy)
[출력]

https://dev-with-gpt.tistory.com/56
네이버클라우드 2주차 딥러닝 과제 미니프로젝트 csv 파일로 NAN, Object, HeatMap 출력하기
[#1. 코드] import numpy as np import pandas as pd from keras.models import Sequential from keras.layers import Dense from sklearn.model_selection import train_test_split from sklearn.metrics import r2_score #1. 데이터 path = './' datasets = pd.read_cs
dev-with-gpt.tistory.com
[#1. 코드]
import numpy as np
import pandas as pd
from keras.models import Sequential
from keras.layers import Dense
from sklearn.model_selection import train_test_split
from sklearn.metrics import r2_score
#1. 데이터
path = './'
datasets = pd.read_csv(path + 'train.csv')
print(datasets.columns)
#print(datasets.head(7))
[출력결과]

[#2. 코드]
x = datasets[['id', 'date', 'bus_route_id', 'in_out', 'station_code', 'station_name',
'latitude', 'longitude', '6~7_ride', '7~8_ride', '8~9_ride',
'9~10_ride', '10~11_ride', '11~12_ride', '6~7_takeoff', '7~8_takeoff',
'8~9_takeoff', '9~10_takeoff', '10~11_takeoff', '11~12_takeoff',
'18~20_ride']]
y = datasets[['18~20_ride']]
print(x)
[출력결과]
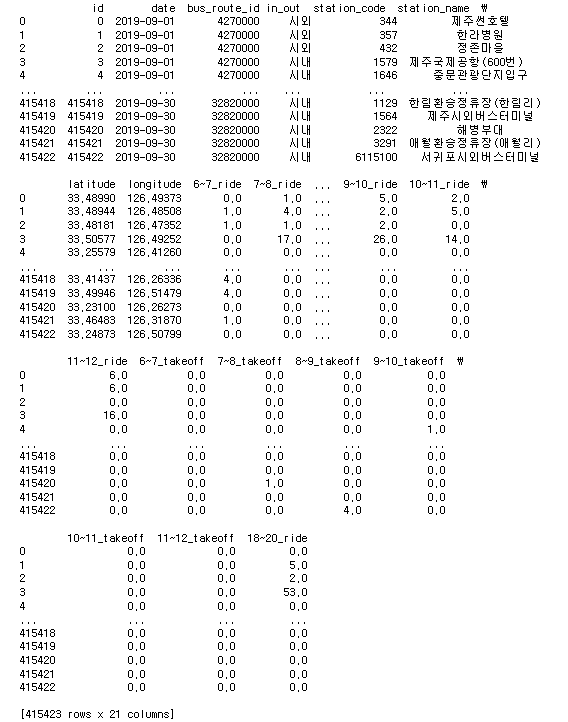
[#3. 코드]
datasets['NAN'] = np.nan #NAN 값 바꿔주기
print(datasets) #출력
print(x)
[출력결과]
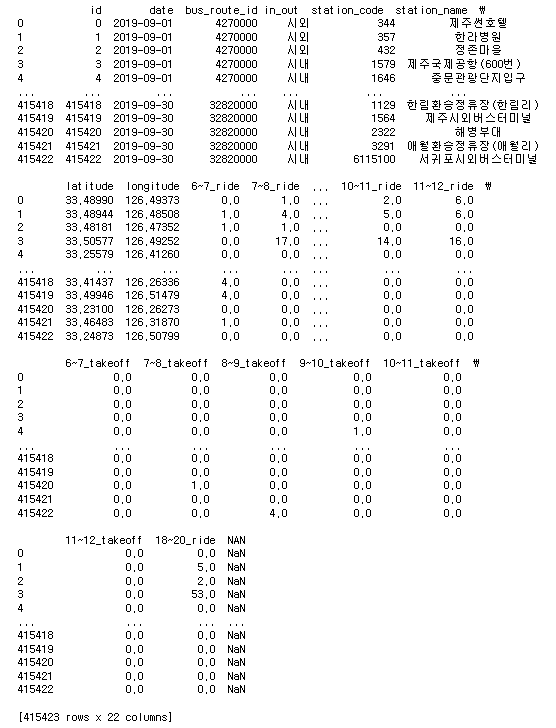
[#4. 코드] 과제1. NAN 값 바꿔주기
datasets['NAN'] = np.nan #NAN 값 바꿔주기
print(datasets) #출력
print(x)
[출력결과]
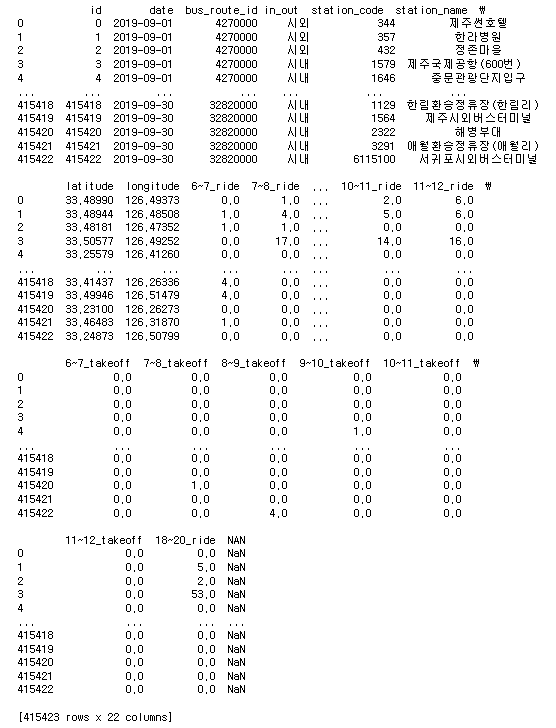
[#5. 코드]
print(x.info()) # info() 컬럼명, null값, 데이터 타입 확인
[출력결과]

[#6. 코드] 과제2. Object 값 바꿔주기
# 문자를 숫자로 변경
from sklearn.preprocessing import LabelEncoder
ob_col = list(x.dtypes[x.dtypes=='object'].index) # object 컬럼 리스트 추출
for col in ob_col :
x[col] = LabelEncoder().fit_transform(x[col].values)
datasets[col]
[출력결과]

[#7. 코드] 과제3. HeatMap 띄우기
#상관계수 히트맵
import matplotlib.pyplot as plt
import seaborn as sns
x_train, x_test, y_train, y_test = train_test_split(
x, y, test_size = 0.2, shuffle=True, random_state=77
)
print(x_train.shape, y_train.shape)
print(x_test.shape, y_test.shape)
sns.set(font_scale = 1.2)
sns.set(rc = {'figure.figsize':(20, 15)})
sns.heatmap(data=datasets.corr(),
square = True,
annot = True,
cbar = True,
cmap = 'coolwarm'
)
plt.show()
[출력결과]
